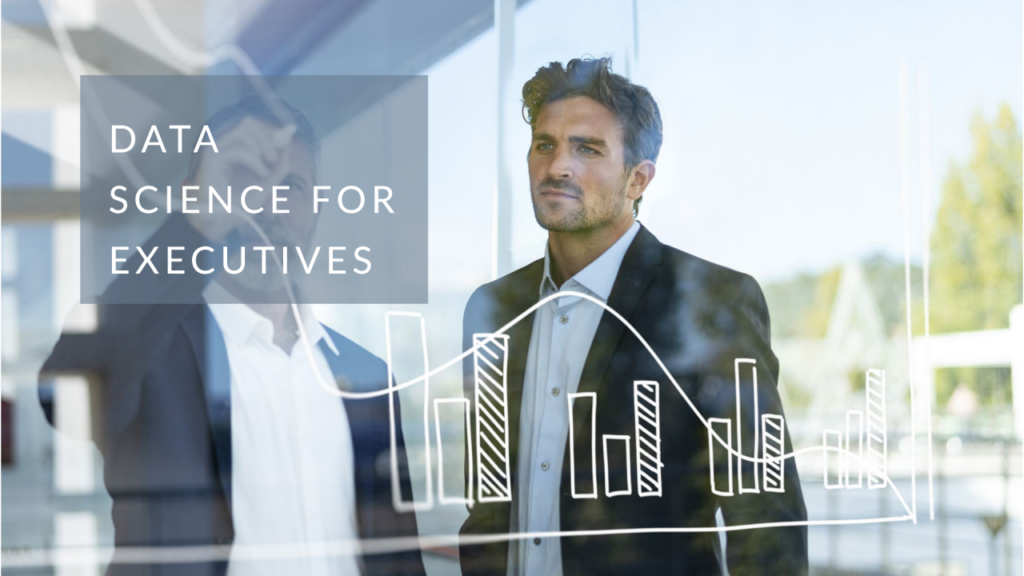
Introduction
In today’s digital age, data science has emerged as a cornerstone for strategic decision-making across industries. For executives, understanding data science is not merely about keeping up with technological trends; it is about leveraging vast amounts of data to drive business growth, optimize operations, and gain a competitive edge. This article delves into the key insights of data science that every executive should be aware of, covering its foundational concepts, practical applications, and the transformative potential it holds for organizations.
Understanding Data Science
What is Data Science?
Data science is an interdisciplinary field that uses scientific methods, algorithms, and systems to extract knowledge and insights from structured and unstructured data. It combines aspects of statistics, computer science, and domain expertise to analyze and interpret complex data sets. In the healthcare industry, data science plays a critical role in improving patient outcomes through predictive analytics and personalized medicine. It enhances operational efficiency in healthcare facilities by optimizing resource allocation and streamlining administrative processes. At its core, data science involves data collection, cleaning, analysis, visualization, and the deployment of data-driven solutions.
The Data Science Workflow
The data science workflow typically consists of several key steps:
1. Data Collection
Data collection is the first step in the data science workflow, involving the aggregation of raw data from various sources. This can include internal databases, external APIs, sensors, and third-party data providers.
2. Data Cleaning
Data cleaning involves processing the collected data to remove inaccuracies, duplicates, and inconsistencies, ensuring high-quality data. This step is crucial as poor data quality can lead to incorrect insights and unreliable models.
3. Exploratory Data Analysis (EDA)
Exploratory Data Analysis (EDA) is the process of examining the data to uncover patterns, relationships, and anomalies. This involves generating statistical summaries and visualizations such as histograms, scatter plots, and box plots.
4. Model Building
Model building is the phase where data scientists develop predictive or descriptive models using machine learning algorithms. This involves selecting suitable algorithms, training them on the cleaned and prepared data, and tuning hyperparameters to optimize performance.
5. Evaluation
Evaluation is the process of assessing the performance and accuracy of the developed models using appropriate metrics. Common metrics include accuracy, precision, recall, F1 score, and mean squared error, depending on whether the problem is classification or regression.
6. Deployment
Deployment involves integrating the trained model into business processes and systems for real-time or batch predictions. This may include embedding the model in a web application, an API, or an automated workflow.
7. Monitoring and Maintenance
Monitoring and maintenance are ongoing activities that involve tracking the model’s performance in a live environment and making necessary updates. This includes detecting drifts in data patterns, retraining the model with new data, and adjusting parameters to maintain accuracy.
The Role of Data Science in Business
Strategic Decision-Making
Executives rely on data science to make informed strategic decisions. By analyzing historical data and identifying trends, data scientists can provide predictive insights that help executives anticipate market shifts, customer preferences, and operational challenges. For example, a retail company might use data science to forecast demand for certain products, enabling better inventory management and marketing strategies. Similarly, in the healthcare industry, data science integrated into care management software can enhance patient care by predicting health outcomes and optimizing treatment plans, ensuring efficient resource utilization and improved patient experiences.
Operational Efficiency
Data science can streamline operations by identifying inefficiencies and optimizing processes. In manufacturing, predictive maintenance models can foresee equipment failures, reducing downtime and maintenance costs. In logistics, route optimization algorithms can minimize delivery times and fuel consumption. These applications demonstrate how data science can enhance productivity and reduce operational expenses.
Customer Insights and Personalization
Understanding customer behavior is crucial for any business. Data science enables companies to analyze customer data to uncover insights about purchasing patterns, preferences, and feedback. This information can be used to personalize marketing efforts, improve customer service, and develop products that better meet customer needs. For instance, e-commerce platforms use recommendation systems powered by data science to suggest products that are likely to interest individual users, thereby increasing sales and customer satisfaction.
Risk Management
Risk management is another area where data science proves invaluable. Financial institutions, for example, use data science to detect fraudulent activities, assess credit risks, and comply with regulatory requirements. By analyzing transaction data and identifying anomalies, these institutions can prevent fraud and minimize financial losses.
Key Technologies in Data Science
Machine Learning
Machine learning, a subset of artificial intelligence, involves training algorithms to make predictions or decisions based on data. It includes supervised learning (e.g., regression, classification), unsupervised learning (e.g., clustering, dimensionality reduction), and reinforcement learning. Executives should understand the basics of machine learning, as it is widely used in predictive analytics, natural language processing, and image recognition.
Big Data Technologies
The term “big data” refers to large and complex data sets that traditional data processing tools cannot handle. Big data technologies, such as Hadoop, Spark, and NoSQL databases, enable the storage, processing, and analysis of massive amounts of data. These technologies are essential for organizations dealing with high-volume data streams, such as social media platforms and IoT devices.
Data Visualization Tools
Data visualization is a crucial aspect of data science, as it helps communicate insights in an understandable and actionable way. Tools like Tableau, Power BI, and D3.js allow data scientists to create interactive and intuitive visualizations. Executives should be familiar with these tools to effectively interpret data and make informed decisions.
Building a Data-Driven Culture
Leadership and Vision
For data science initiatives to succeed, executive leadership must foster a data-driven culture within the organization. This involves setting a clear vision for how data science can drive business value, allocating resources for data projects, and promoting a mindset that values data-driven decision-making across all levels of the organization.
Talent Acquisition and Development
Hiring skilled data scientists, analysts, and engineers is critical for building a robust data science team. Additionally, investing in continuous training and development helps keep the team updated with the latest tools and techniques. Executives should also consider upskilling other employees to enhance their data literacy, enabling them to leverage data in their daily tasks.
Data Governance and Ethics
As organizations collect and analyze more data, issues related to data privacy, security, and ethics become increasingly important. Executives must establish strong data governance frameworks to ensure data quality, compliance with regulations, and ethical use of data. This includes implementing policies for data access, anonymization, and consent management. For example, medical coding companies must adhere to strict privacy standards to protect patient information while accurately coding medical records, ensuring both regulatory compliance and ethical handling of sensitive data.
Case Studies: Data Science in Action
Netflix: Personalized Content Recommendations
Netflix is a prime example of a company that uses data science to drive its business. The streaming giant leverages machine learning algorithms to analyze user behavior and preferences, providing personalized content recommendations. This approach not only enhances user experience but also helps Netflix retain subscribers and increase engagement.
Walmart: Inventory Optimization
Walmart utilizes big data analytics to optimize its inventory management. By analyzing sales data, weather patterns, and social media trends, Walmart can predict product demand more accurately and ensure that its shelves are stocked with the right products at the right time. This reduces inventory costs and improves customer satisfaction.
Tesla: Autonomous Driving
Tesla’s autonomous driving technology relies heavily on data science and machine learning. The company collects vast amounts of data from its fleet of vehicles, which is then used to train algorithms that improve the performance of its self-driving systems. This continuous learning loop enables Tesla to enhance safety and efficiency in its vehicles.
Overcoming Challenges in Data Science Implementation
Data Quality and Integration
One of the main challenges in data science is ensuring data quality and integration. Inconsistent, incomplete, or siloed data can hinder the effectiveness of data science projects. Executives need to invest in data management tools and practices that ensure clean, integrated, and accessible data across the organization.
Bridging the Gap Between Data Science and Business
Another common challenge is bridging the gap between data science teams and business stakeholders. Effective communication and collaboration are essential to ensure that data science initiatives align with business goals and deliver tangible value. Executives can facilitate this by promoting cross-functional teams and encouraging data scientists to develop a deep understanding of business processes.
Scalability and Flexibility
As data science projects grow, scalability and flexibility become crucial. Organizations need to adopt scalable architectures and cloud-based solutions that can handle increasing data volumes and computational demands. Flexibility in choosing the right tools and technologies is also important to adapt to evolving business needs and technological advancements.
Future Trends in Data Science
Artificial Intelligence and Deep Learning
Artificial intelligence (AI) and deep learning are poised to revolutionize data science. AI technologies are becoming more sophisticated, enabling advanced applications such as natural language processing, image recognition, and autonomous systems. Executives should stay informed about AI developments and consider how these technologies can be integrated into their data science strategies.
Augmented Analytics
Augmented analytics leverages AI and machine learning to automate data preparation, insight generation, and explanation. This trend aims to make data science more accessible to non-experts, enabling executives and other business users to perform complex analyses with minimal technical knowledge. Tools that support augmented analytics can democratize data science and foster a more data-driven culture.
Data Privacy and Security
With increasing concerns about data privacy and security, regulatory frameworks like GDPR and CCPA are becoming more stringent. Executives must stay abreast of these regulations and ensure that their data practices comply with legal requirements. Investing in advanced security measures and privacy-preserving techniques, such as differential privacy and federated learning, can help protect sensitive data while enabling data-driven innovation.
Conclusion
Data science offers transformative potential for businesses, enabling more informed decision-making, operational efficiency, personalized customer experiences, and effective risk management. For executives, understanding the key concepts, technologies, and challenges of data science is crucial for leveraging its full potential. By fostering a data-driven culture, investing in talent and technology, and staying informed about emerging trends, executives can harness the power of data science to drive business success in the digital age.