In the realm of data science, the journey from raw data to actionable insights is often complex and multifaceted. Traditional approaches rely heavily on individual expertise and predefined algorithms to navigate through this labyrinth of data. However, with the advent of swarm intelligence, a new paradigm is emerging—one that leverages the collective behavior of decentralized systems to tackle data exploration and analysis collaboratively. In this article, we delve into the concept of collaborative data exploration and how swarm intelligence fosters collective learning within the realm of data science.
Understanding Swarm Intelligence
Swarm intelligence draws inspiration from the collective behavior of social organisms in nature, such as ant colonies, bird flocks, and bee swarms. These natural systems exhibit emergent intelligence, where complex, adaptive behaviors arise from the interactions of simple individuals following local rules. By mimicking such decentralized, self-organizing principles, swarm intelligence algorithms aim to solve complex problems more efficiently and robustly than traditional methods.
So, in today’s dynamic landscape of data science and technology, hiring a developer with knowledge of swarm intelligence algorithms can significantly enhance the innovation and problem-solving capabilities of your team. For finding such talent, platforms like Lemon.io offer access to skilled developers proficient in swarm technologies at https://lemon.io/tech-stacks/swarm/.
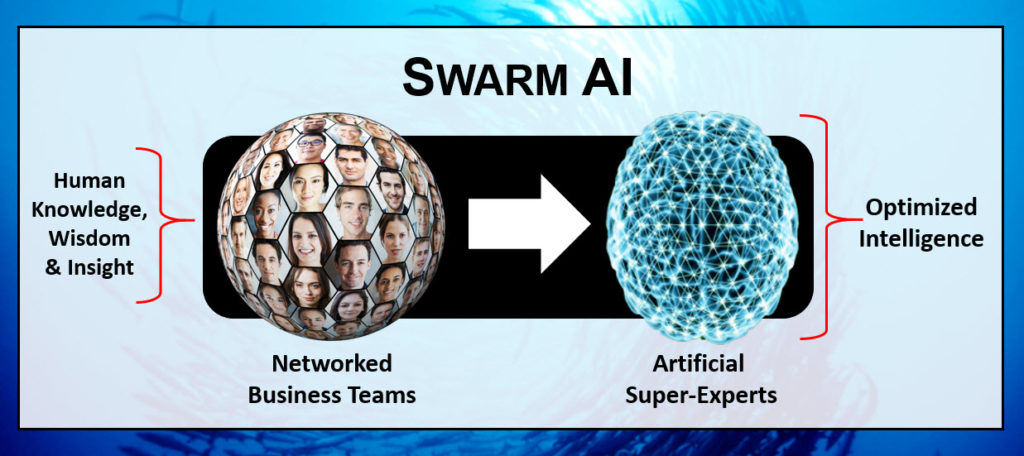
The Role of Swarm Intelligence in Data Exploration
Data exploration is a fundamental step in the data analysis pipeline, involving the discovery, visualization, and understanding of patterns, trends, and anomalies within datasets. Traditionally, this process heavily relies on the expertise of data scientists and predefined analytical techniques. However, with the application of swarm intelligence, data exploration becomes a collaborative endeavor where multiple agents, each representing a unique perspective or approach, interact and collectively navigate through the data landscape.
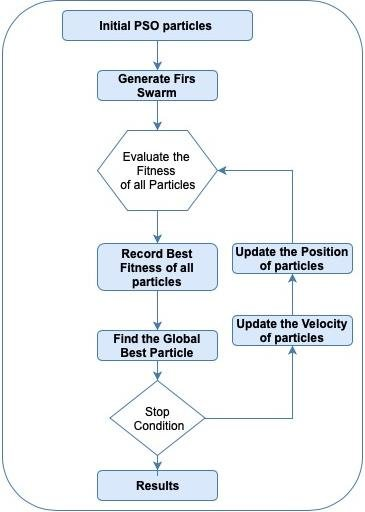
Collective Learning through Swarm Intelligence
At the heart of swarm intelligence lies the concept of collective learning—the ability of a swarm to adapt, evolve, and improve its performance over time through the accumulation and exchange of knowledge. In the context of data exploration, this translates to the swarm collectively gaining insights, refining strategies, and enhancing its ability to uncover hidden patterns and correlations within the data.
One example of collaborative data exploration using swarm intelligence is the application of particle swarm optimization (PSO) algorithms. In PSO, a population of particles iteratively explores the search space by adjusting their positions based on their individual experiences and the shared knowledge of the swarm. This collaborative exploration enables PSO to efficiently converge towards optimal solutions in complex, high-dimensional datasets.
Advantages of Collaborative Data Exploration with Swarm Intelligence
- Distributed Computation: Swarm intelligence algorithms leverage parallelism and distributed computation, allowing multiple agents to explore different regions of the data simultaneously. This parallel exploration accelerates the discovery process and enables scalability to large datasets.
- Adaptability: Swarm intelligence systems exhibit adaptive behavior, dynamically adjusting their strategies in response to changes in the data landscape. This adaptability ensures robustness and resilience in the face of noisy or evolving datasets.
- Exploration of Diverse Perspectives: By incorporating diverse agents with different viewpoints or exploration strategies, swarm intelligence facilitates the exploration of a broader range of hypotheses and patterns within the data. This diversity enhances the robustness of the exploration process and mitigates the risk of algorithmic biases.
- Incremental Learning: Swarm intelligence algorithms support incremental learning, where the swarm continuously integrates new data and updates its internal state. This iterative learning process enables the swarm to refine its understanding of the data over time and adapt to evolving patterns or trends.
Challenges and Considerations
Despite its potential benefits, collaborative data exploration with swarm intelligence poses several challenges and considerations:
- Scalability: While swarm intelligence algorithms offer scalability advantages, managing large swarms and coordinating their interactions can become computationally expensive, particularly for massive datasets.
- Complexity of Interactions: The dynamics of swarm interactions can be highly complex, making it challenging to understand and interpret the collective behavior of the swarm. Ensuring transparency and interpretability in swarm-based data exploration is essential for building trust in the results.
- Integration with Human Expertise: Effective collaboration between swarm intelligence systems and human experts requires thoughtful design and integration. Balancing the autonomy of the swarm with human guidance and oversight is crucial for achieving synergistic outcomes.
Future Directions and Applications
The synergy between swarm intelligence and data exploration opens up exciting avenues for future research and applications. Some potential directions include:
- Hybrid Approaches: Integrating swarm intelligence with other machine learning techniques, such as deep learning or reinforcement learning, to enhance the exploration and exploitation capabilities of the swarm.
- Domain-Specific Applications: Tailoring swarm intelligence algorithms to specific domains or industries, such as healthcare, finance, or cybersecurity, to address domain-specific challenges and opportunities.
- Human-Swarm Collaboration: Exploring novel interfaces and interaction paradigms to facilitate seamless collaboration between human analysts and swarm intelligence systems, leveraging the complementary strengths of both.
Final Words
Collaborative data exploration with swarm intelligence represents a paradigm shift in how we approach the analysis and interpretation of complex datasets. By harnessing the collective intelligence of decentralized systems, swarm intelligence algorithms offer a powerful framework for uncovering hidden insights, patterns, and relationships within data. As we continue to explore and refine these techniques, the potential for collective learning and discovery in data science remains vast, promising new insights and innovations across a wide range of domains.
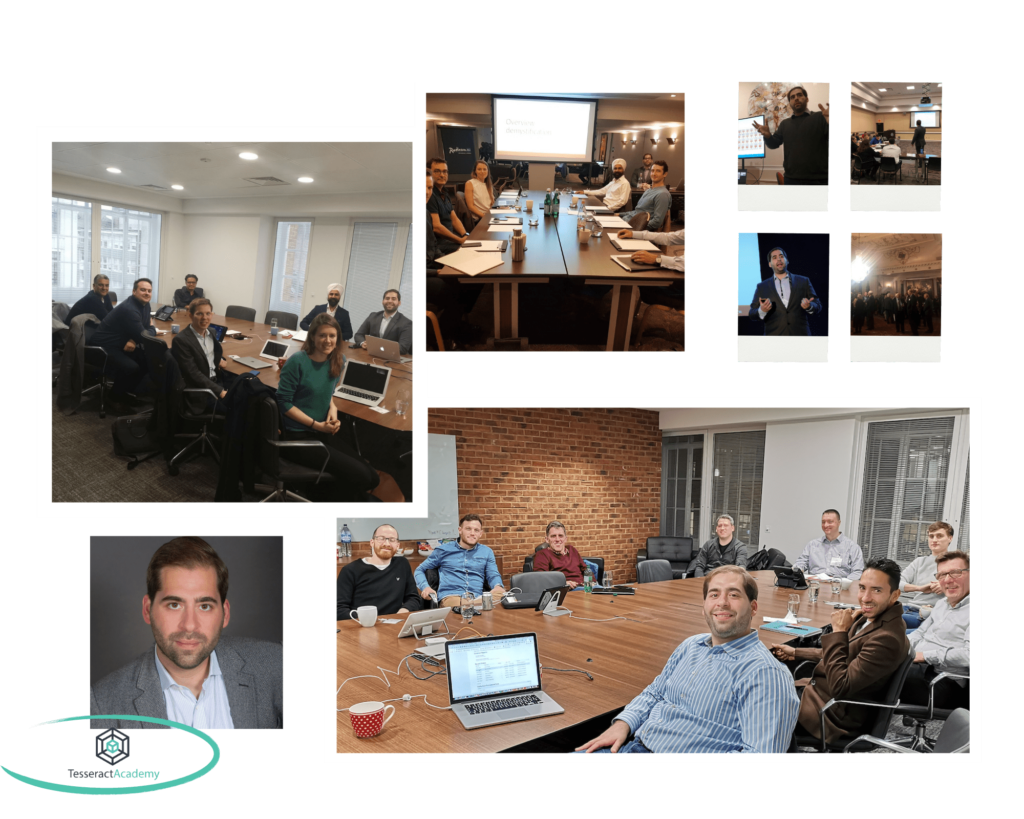