We live in the era of personalisation. Through data science it becomes possible to suggest, discover and create products that are tailor-suited to each individual’s preferences.
The first wave of personalisation through data science came in the form of recommender systems. Recommendation engines predict what you are going to like, increasing the profit margin. Amazon uses a recommender system to predict what products you are most likely to buy. Netflix uses a recommender system to suggest movies, and Spotify uses a recommender system to come up with playlists.
Recommendations, however, are somewhat static. A recommender simply suggests products, and the user can choose to buy them or not. There are further optimisations we can do through data science in order to offer a more personalised service. One such approach is dynamic pricing.
[sc_fs_faq sc_id=”fs_faqi2tnk4jgo” html=”true” headline=”h3″ img=”” question=”What is dynamic pricing?” img_alt=”” css_class=”” ]Dynamic pricing creates different prices for different customers and circumstances. The first example of dynamic pricing was the creation of multiple ticket types of American Airlines in the 1980s. American Airlines was losing ground to budget airlines which had just appeared in the market. They figured out that not all customers are the same, some mostly caring about getting a cheap price, and others caring about a good service. The solution they came up with was to offer different ticket types, from economy to business. This is now common practice in all airlines, as well as in other types of industries, like concerts.[/sc_fs_faq]
Dynamic pricing has advanced a lot since then. In theory, the idea behind dynamic pricing is that each person has a different price elasticity. This can depend on the individual, but also on the individual’s circumstances.
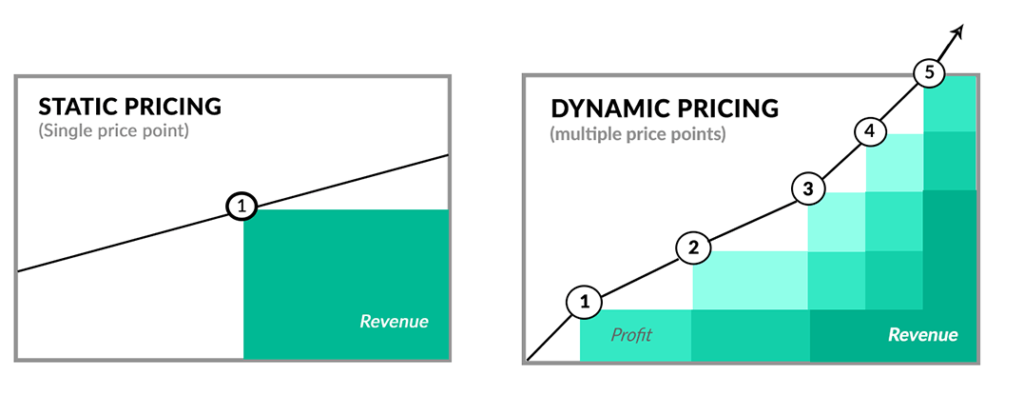
One of the most famous applications of dynamic pricing is Uber’s surge pricing. At times of high demand, Uber will increase prices in order to bring more drivers on the road. Obviously, this has the effect of reducing waiting times, but it can also cause issues, like for this person, that had to pay $14000 for a 20-minute ride.
There are other types of dynamic pricing besides surge pricing. Another way is to come up with unique discounts or product bundles for each user. Or to provide some users with a completely customised offers for short periods in time. In one way or another, dynamic pricing is a prediction problem, and this makes machine learning our best tool to tackle it.
The general approach for creating a dynamic pricing model is the following:
- Decide on the level of granularity you are aiming for. Do you care about modelling the individual user, groups of users (e.g. specific types of customers), or the whole user base?
- Build a model to predict whether someone will make a purchase (or the total number of purchases), based on the different parameters.
- Use an optimisation algorithm to discover the optimal price and product features, in order to maximise the proability of purchasing. This method can also be used for creating product bundles and discounts.
The last step in the method is something I call the “predict and optimise framework”. It was also discussed in video by the Tesseract Academy which you can find below:
If you want to learn more about surge pricing, make sure to also check out the video by the Tesseract Academy posted previously, where we talk about different ways to use machine learning for dynamic pricing.