In recent years, the field of data science has become increasingly important in decision-making processes across various industries. With the rise of algorithmic decision-making, however, concerns about bias and fairness have emerged, making it one of the most informative topics to write about, especially for students. So, it is essential to address these ethical considerations to ensure that data-driven decisions are unbiased and fair.
Defining Ethics in the Context of Data Science
Ethics in data science refers to the principles and guidelines that govern the responsible conduct of data analysis and decision-making. It encompasses respect for privacy, transparency in data collection and, use accountability for the consequences of decisions, and fairness in the treatment of individuals.
Privacy concerns are at the forefront of ethical considerations in data science, as the misuse or unauthorized access to personal data can have serious implications for individuals. Data scientists must prioritize the protection of sensitive information and implement robust security measures to prevent breaches that could compromise privacy.
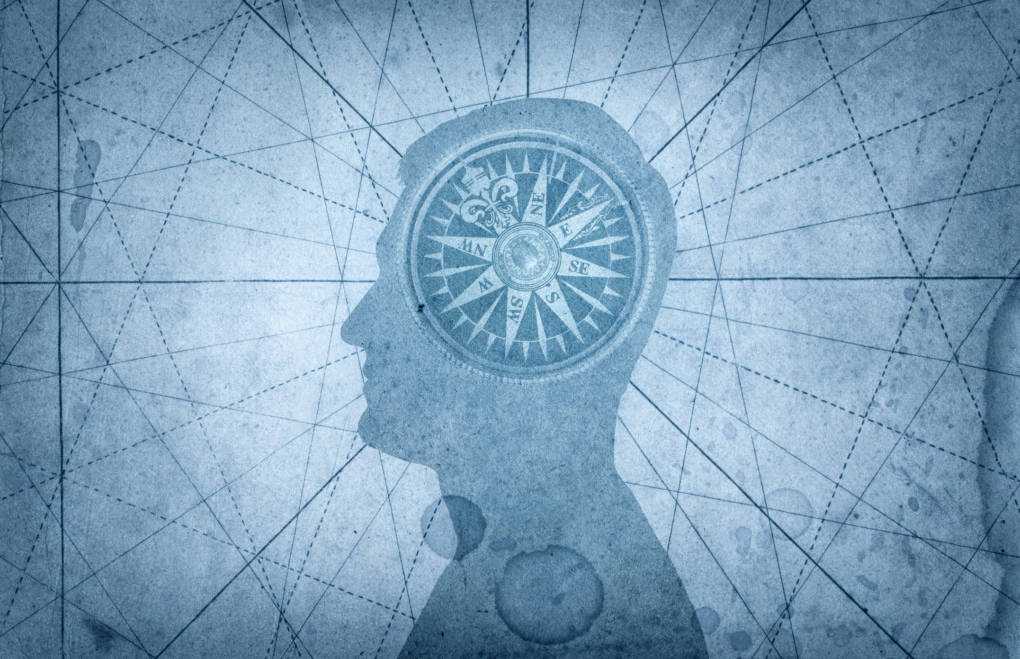
The Role of Data Scientists in Upholding Ethical Standards
Data scientists have a unique responsibility in upholding ethical standards in their work. They should actively consider the potential biases and ethical implications of the data they use and the algorithms they develop. Data scientists should prioritize fairness, transparency, and accountability throughout the entire data science process.
Moreover, data scientists should engage in ongoing ethical reflection and dialogue within the field to address emerging challenges and dilemmas. By fostering a culture of ethical awareness and responsibility, data scientists can contribute to the development of best practices that benefit society as a whole.
The Intersection of Bias and Fairness in Algorithmic Decision Making
Bias in algorithmic decision-making occurs when the decisions made by algorithms result in disproportionate outcomes for certain groups of people. This bias can be unintentional but can perpetuate and amplify existing societal inequalities.
Algorithmic decision-making processes have become increasingly prevalent in various aspects of our lives, from determining credit scores to influencing judicial decisions. However, the reliance on these algorithms raises concerns about the potential biases that may be embedded within them. These biases can stem from the data used to train the algorithms, as well as the design and implementation of the algorithms themselves.
Identifying Bias in Data and Algorithms
The first step in addressing bias is to identify it. Data scientists must critically examine the data being used, as it can contain inherent biases that unfairly disadvantage certain groups. Additionally, algorithms must be scrutinized for potential biases that may be embedded within them.
Moreover, the interpretation of data and the design of algorithms are not immune to human biases. The choices made in selecting features, defining success metrics, and setting thresholds can inadvertently introduce or perpetuate biases. It is crucial for developers and data scientists to be aware of these potential biases and actively work to mitigate them.
The Impact of Bias on Fairness in Decision Making
Bias in algorithmic decision-making can lead to unfair treatment of individuals or groups and perpetuate societal inequities. It can result in discriminatory outcomes in areas such as hiring, lending, criminal justice, and healthcare. It is essential to address bias to ensure fairness in algorithmic decision-making.
Fairness in algorithmic decision-making is not just a technical challenge but also a moral and ethical one. The consequences of biased algorithms can have far-reaching effects on individuals and communities, reinforcing systemic inequalities and hindering progress toward a more just society. By acknowledging and actively combating bias in algorithmic decision-making, we can strive toward creating more equitable and just systems for all.
Strategies for Addressing Bias in Data Science
While bias in data science can be challenging to eliminate entirely, there are strategies that can help mitigate its effects and promote fairness.
Data scientists can employ techniques to detect and measure bias in datasets and algorithms. By analyzing the demographic composition of the data and the outcomes of the algorithms for different groups, biases can be identified and quantified.
Once biases are identified, data scientists must take necessary steps to mitigate them. This may involve adjusting the training data to be more representative, modifying algorithms to account for fairness, or developing new algorithms altogether.
Ensuring Fairness in Algorithmic Decision Making
Apart from tackling bias, ensuring fairness in algorithmic decision-making is essential to prevent discriminatory results.
Data scientists should follow principles that champion fairness, like treating similar cases equally and steering clear of unjust discrimination. Fair decision-making hinges on transparency and accountability every step of the way.
Methods such as ensuring consistent performance across diverse groups, tweaking decisions to eliminate biases, and offering explanations for algorithmic decisions can foster fairness in algorithmic decision-making.
The Future of Ethics in Data Science
Looking ahead to the future of ethics in data science, as the field progresses, fresh ethical dilemmas will emerge. Emerging technologies like artificial intelligence and machine learning introduce distinctive ethical concerns. Matters like safeguarding data privacy, ensuring transparency in algorithms, and grappling with the ethics of automation will demand continual scrutiny and oversight.
Regulatory structures and ethical principles are pivotal in guaranteeing ethical conduct in data science. It’s imperative for government bodies, professional organizations, and industry alliances to work together to set benchmarks that prioritize fairness, privacy, and responsibility.
Final Words
To wrap up, ethical concerns in data science play a vital role in tackling bias and championing fairness in algorithmic decision-making. It’s on data scientists to grasp and tackle bias in their data and algorithms, putting fairness and accountability at the forefront of every step in the data science journey. By deploying methods to spot and lessen bias and advocating for fairness in decision-making, data scientists pave the way for a more just and ethical future in the field.
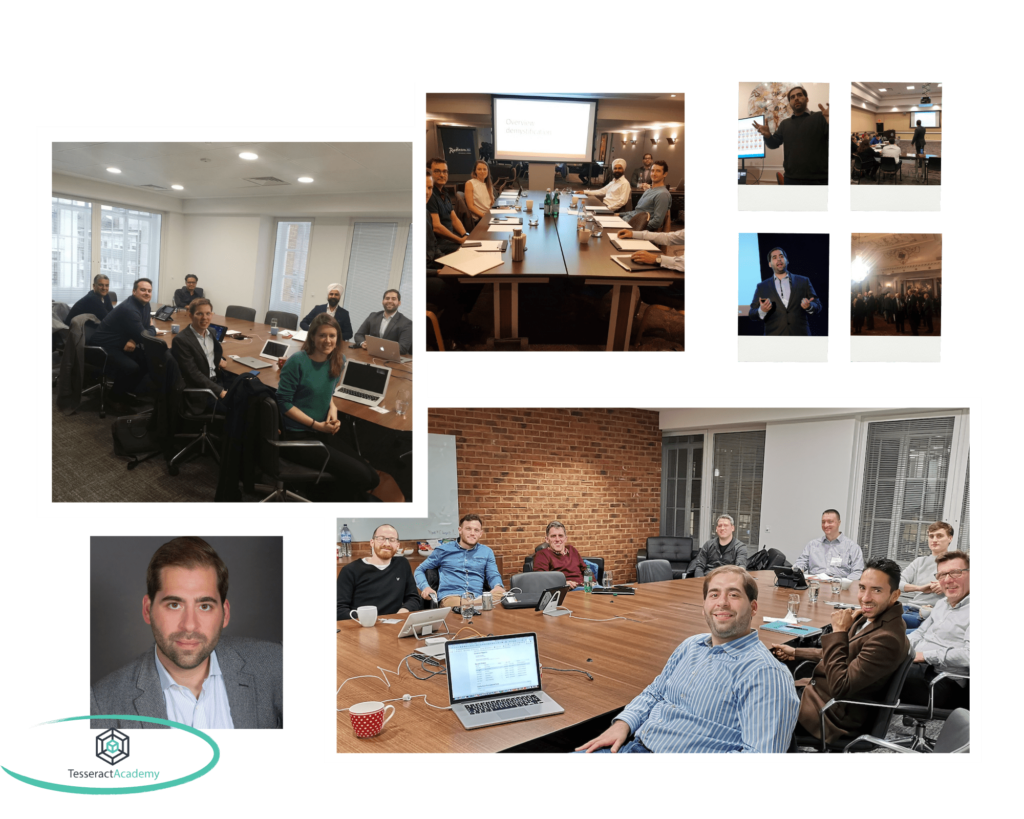
Unlock the power of data science & AI with Tesseract Academy! Dive into our data science & AI courses to elevate your skills and discover endless possibilities in this new era.
- Unlock the Power of AI: Unveiling Innovative Ways to Earn Income Online
- How Database Monitoring Services Can Ensure Business Continuity
- Artificial Intelligence in SEO: Exploring Opportunities for Semantic SEO, Data-Driven Strategies, Link Building, and More
- New book is out! Business models in emerging technologies