Artificial Intelligence (AI) is currently experiencing a significant resurgence, particularly in the field of text generation. Historically, AI in this area relied on basic sentence structures, but recent advancements have led to the development of more complex and context-aware outputs.
A key factor in this transformation is retrieval augmented generation (RAG). This innovative approach combines the capabilities of information retrieval with generative AI models, enhancing their functionality. According to a 2023 McKinsey survey, 40% of respondents indicated their organizations plan to increase AI investment, largely influenced by advancements in general AI technologies like RAG.
In this post we aim to clarify the role of retrieval augmented generation in AI, exploring its mechanisms, implications, and the significant impact it has on reshaping online text generation. We will explore how RAG not only improves language skills but also revolutionizes the broader fields of machine learning and natural language processing.
Understanding Text Generation in AI
Traditionally, in the AI domain, text generation models majorly focussed on being able to generate coherent and contextually relevant language outputs primarily based on pre-trained data and algorithms.
However, often these models grappled with incidents such as lack of context, relevance, and some other times, factual inaccuracies too. Retrieval-augmented approaches dawned with a ray of hope in overcoming these drawbacks, through some additional layer of external information retrieval into the generative process.
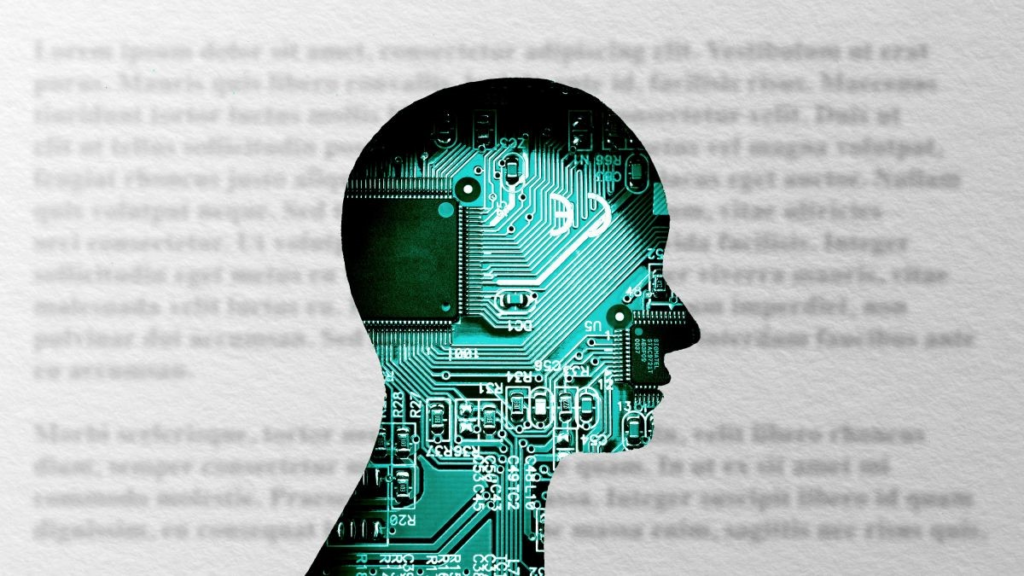
The Mechanics of Retrieval Augmented Generation
RAG stands distinct from its predecessors, blending the retrieval of external data with generative mechanisms to produce more accurate and context-rich text outputs. At its core, RAG operates through two primary components: a retrieval component and a generation component.
The retrieval component is like a researcher, meticulously sourcing relevant information from a vast expanse of data. This information is then seamlessly integrated by the generation component, which is responsible for weaving this external data into coherent and contextually appropriate text. This intricate process of integration allows RAG to elevate the standard of AI-generated text by making it more informed and contextually grounded.
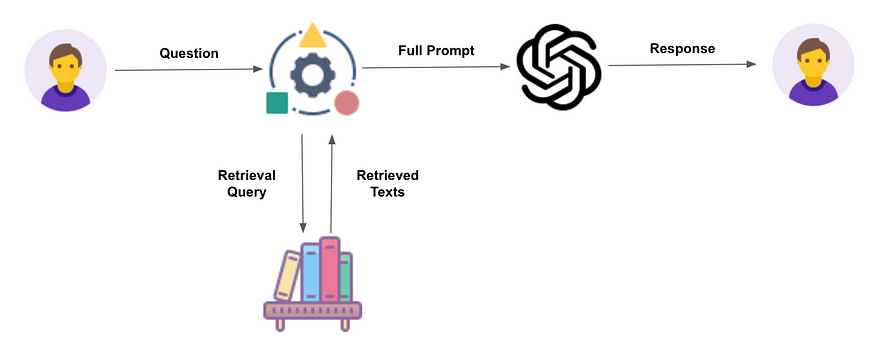
The Advantages of Retrieval Augmented Generation
The advantages of RAG are multifaceted. Firstly, it significantly enhances the accuracy of generated text. By pulling in relevant and often real-time information, RAG ensures that the text is not only contextually appropriate but also factually correct. This characteristic is particularly beneficial in domains where precision and up-to-date information are crucial.
RAG also exhibits impressive context awareness. Unlike traditional models that all too often operate in a vacuum, RAG may incorporate external context and provide more grounded and comprehensive responses. Moreover, its versatility extends not only to creative but also to technical documentation that expands the AI in text generation.
Challenges and Considerations in RAG
Despite its numerous benefits, RAG is not without its challenges. One primary concern is balancing the retrieval and generation aspects. Fine-tuning a RAG system to ensure that it retrieves relevant information without compromising the fluidity and originality of the generated text is a complex task.
Additionally, RAG systems are not immune to the pitfalls of misinformation and biases present in the source data. This necessitates robust filtering mechanisms to ensure the reliability of retrieved information. Moreover, ethical considerations are paramount, as the responsible use of RAG in generating content that is fair and unbiased is a critical aspect of its deployment.
The Future of RAG in AI Text Generation
Looking ahead, the potential developments in RAG technology are bound to shape the future of text generation in AI. Continuous improvements in both retrieval accuracy and generative capabilities are expected, which will further enhance the efficacy and reliability of RAG systems.
Furthermore, with the consistent and ever-increasing involvement of AI in different sectors, RAG is supposed to take on a newer, more central, and diverse role in both processing as well as writing text. The implications are huge, and they suggest a future in which AI will be capable not only of answering questions for us but also of assisting in the composition of complex, creative text as well – thus further reducing the gap between man and machine.
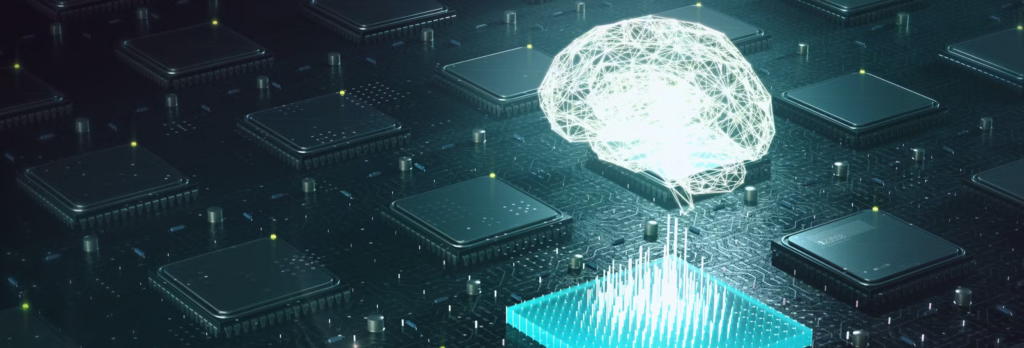
Final Thoughts
Retrieval augmented generation is a significant milestone in the journey of AI’s capabilities in text generation. RAG is truly remarkable as it has resolved the constraints in the traditional model by having retrieval of external information and procedures for generation.
In other words, the possible future implications of AI applications in various sectors would be promising and exuberating as we discover and improvise further developments in this technology.
The RAG integration into more sophisticated AI systems heralds a future where the bounds of machine-generated text are continually being expanded – offering a glimpse into the immense potential of AI in terms of mimicking and augmenting human cognitive processes.