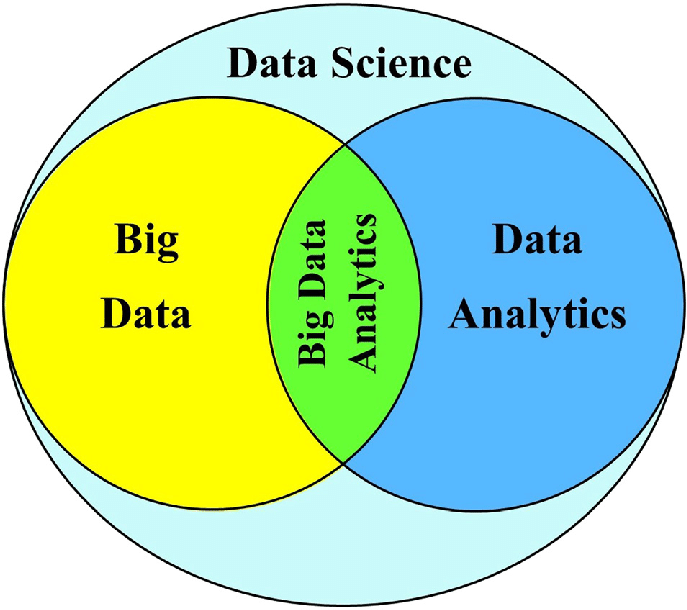
In the contemporary era of technology, the concept of Big Data has become pivotal. It refers to the massive volumes of structured and unstructured data that inundate businesses daily. Big Data is not merely about the data’s size; it’s more about what organizations do with the data. Data Science plays a crucial role here, using scientific methods, processes, algorithms, and systems to extract knowledge and insights from these large data sets.
The aim of this discussion is to explore how Big Data has impacted the daily practices of data scientists, reshaping the way they collect, analyze, and interpret data, and consequently, influence business strategies and decision-making processes.
Evolution of Data Science with Big Data
Historically, data science involved analyzing relatively small datasets using basic statistical tools. However, with the advent of Big Data, the landscape has dramatically transformed. This era is characterized by the ‘Three Vs’:
Volume: The sheer volume of data generated by machines, networks, social media, and people.
Velocity: The unprecedented speed at which this data is generated and processed.
Variety: The increasing types of data (text, audio, video, etc.), often unstructured.
This evolution has necessitated the development of new tools and techniques in data science.
Changes in Data Collection and Storage
The changes in data collection and storage in the era of Big Data are profound. Traditionally, data was often collected manually and stored in structured formats. With Big Data, automated data collection has become the norm, utilizing a myriad of sources like IoT devices, social media, and online transactions. This shift has led to an exponential increase in both the volume and variety of data. Storage solutions have also evolved, moving from localized servers to more scalable and flexible cloud storage solutions and advanced databases like NoSQL, which are designed to handle the size and complexity of Big Data. This evolution is enabling more efficient and effective data management and analysis.
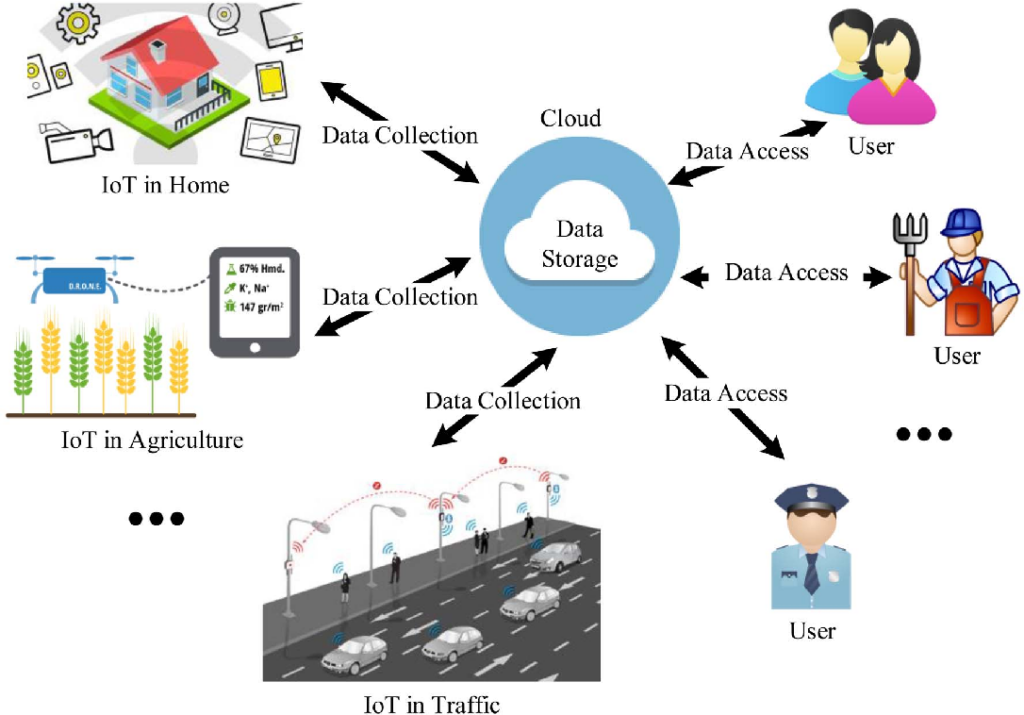
Advanced Analytical Tools and Techniques
The advent of Big Data has necessitated the development of advanced analytical tools and techniques. This includes:
Machine Learning and AI Algorithms: The vast complexity and volume of Big Data have spurred the rapid adoption of AI and machine learning algorithms. These technologies enable more nuanced and predictive analytics, allowing for deeper insights into large and complex datasets.
Big Data Analytics Tools: Tools specifically designed for handling large datasets, like Apache Hadoop and Spark, have become essential in the Big Data landscape. They provide the necessary infrastructure to process and analyze vast amounts of data efficiently.
Data Processing and Management Challenges: The rise of Big Data brings significant challenges in data processing and management. Handling the sheer volume of data requires advanced computational methods and algorithms. Additionally, maintaining data quality and effectively cleaning vast datasets has become more challenging, necessitating more sophisticated and innovative approaches.
These advancements in tools and techniques have fundamentally transformed how data is processed, analyzed, and utilized, leading to more informed decisions and strategies in various industries.
Big Data introduces significant challenges in data processing and management, which include:
Complexity in Data Processing: The large volumes of Big Data demand advanced computational methods and algorithms. Traditional data processing techniques are often insufficient for the scale and complexity of Big Data.
Data Quality and Cleaning: Big Data often includes diverse and unstructured data, raising challenges in maintaining data quality. Cleaning such extensive datasets to ensure accuracy and reliability is more complicated, requiring sophisticated tools and techniques.
Big Data has significantly enhanced decision-making capabilities:
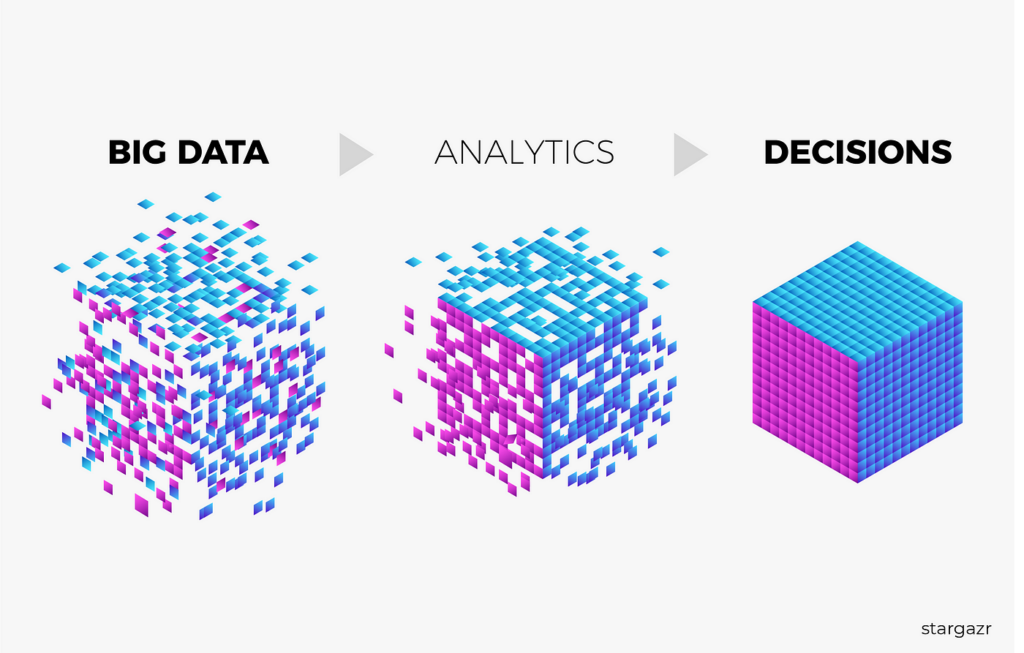
Big Data has greatly enhanced decision-making capabilities in several ways. It enables businesses to analyze vast amounts of information, uncovering patterns and trends that were previously invisible. This deep insight allows for more informed and strategic decisions. Big Data analytics can predict customer behavior, optimize operations, and identify new market opportunities. Essentially, it transforms decision-making from a gut-based approach to a data-driven process, leading to more accurate, efficient, and effective business strategies.
Data-Driven Decision Making: With Big Data, organizations have the ability to analyze extensive datasets, leading to more informed decisions. This analysis helps in understanding complex patterns, trends, and relationships within the data, thereby enabling businesses to make strategic decisions based on concrete data insights rather than intuition or limited information.
Strategic Business Insights: Data scientists, through their analysis of Big Data, provide valuable insights that directly influence business strategies. They play a crucial role in identifying new market opportunities, understanding customer preferences, and suggesting operational improvements. These insights are pivotal in shaping a business’s strategic direction, ensuring that decisions are aligned with market dynamics and consumer behavior.
Ethical Considerations and Privacy Concerns
The era of Big Data raises serious ethical and privacy concerns:
Data Privacy: The massive amount of data collected heightens concerns about individual privacy. Data scientists must ethically handle this data, ensuring privacy and security to maintain public trust and comply with legal standards.
Ethical Use of Data: It’s essential to use data ethically, particularly when it involves sensitive information. This includes ensuring data is used responsibly, avoiding misuse or misinterpretation, and respecting the confidentiality and integrity of the data subjects.
The Future of Data Science with Big Data
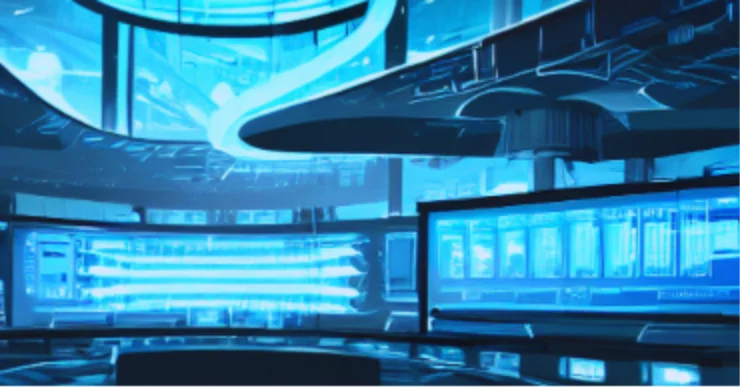
The future of data science in the context of big data is a multifaceted and evolving field, poised to significantly impact various industries and aspects of life. Here are some key aspects to consider:
Advanced Machine Learning and AI Integration: As data volumes grow, the complexity and sophistication of machine learning models will also increase. We can expect more advanced forms of AI, including deep learning and neural networks, to become integral in extracting valuable insights from large datasets.
Real-time Data Processing and Analytics: The ability to process and analyze data in real-time will become increasingly important. This could lead to more dynamic decision-making processes in industries like finance, healthcare, and manufacturing, where immediate insights can be critical.
Enhanced Data Privacy and Security: With the growth of big data, concerns regarding data privacy and security will become more prominent. This will likely drive innovations in secure data processing methods, like federated learning, and stricter data governance and regulatory compliance.
Quantum Computing and Big Data: The potential integration of quantum computing could revolutionize data science. Quantum computers, with their ability to handle immensely complex calculations, could enable new levels of data analysis previously thought impossible.
IoT &Computing: Internet of Things (IoT) producdces large amounts of data. Edge computing, which processes data closer to where it’s generated, will likely become more prevalent. This will reduce latency and reliance on central data processing, enabling faster and more efficient data handling.
Predictive and Prescriptive Analytics: The future of data science will see a shift from descriptive analytics to predictive and prescriptive analytics. This means not just understanding what happened or what is happening, but also predicting future trends and prescribing actions.
Data Democratization: The trend towards making data more accessible and understandable to non-experts will continue. Tools and platforms that simplify data analysis will become more common, allowing more people across an organization to make data-driven decisions.
Cross-Domain Data Integration: The integration of data from various domains and sources will become more seamless. This could lead to more comprehensive insights and the discovery of new correlations between previously unlinked data types.
Ethical and Responsible AI: With AI playing a larger role in data science, ethical considerations will gain prominence. This includes ensuring AI fairness, avoiding biases in machine learning models, and being transparent about AI decision-making processes.
Augmented Analytics: The use of augmented analytics, which combines AI and machine learning to automate data preparation and analysis, will likely grow. This could make complex data analysis more accessible to a broader audience.
Conclusion
The impact of Big Data on the daily roadmap for data scientist and data analytics lifecycle is profound. It has not only transformed the technical aspects of data collection, analysis, and storage but also brought to the fore new ethical and strategic considerations. As we move forward, adapting to these changes is not just beneficial but necessary for organizations to stay competitive and relevant in a data-driven world.